Communication and storytelling in data science refer to the ability to effectively convey insights, findings, and the value of data-driven solutions to both technical and non-technical stakeholders. It involves presenting complex information in a clear, concise, and compelling manner, using visualizations, narratives, and persuasive techniques.
Here are some key aspects of communication and storytelling in data science:
- Simplifying Complex Concepts: Data scientists often work with intricate models, algorithms, and technical jargon. Effective communication involves translating these complex concepts into simple terms that can be understood by a broader audience. This requires the ability to break down complex ideas, use analogies, and avoid unnecessary technical details.
- Visualizations: Visualizations play a crucial role in data science communication. Creating clear and visually appealing graphs, charts, and infographics helps in presenting data patterns, trends, and insights in an intuitive and accessible way. Choosing appropriate visualization techniques and highlighting key information are important for effective communication.
- Tailoring the Message: Different stakeholders may have varying levels of technical knowledge and different interests. It is essential to understand the audience and tailor the message accordingly. Presenting information that is relevant, impactful, and aligned with the stakeholders’ goals and concerns increases the chances of successful communication.
- Storytelling: Stories have a powerful impact on human understanding and engagement. Data scientists can use storytelling techniques to create narratives around data. By framing the problem, describing the process, and highlighting the outcomes and implications, they can captivate the audience and make data-driven insights more relatable and memorable.
- Contextualizing Results: Providing context is crucial for interpreting and understanding data-driven findings. Explaining the limitations, assumptions, and uncertainties associated with the analysis helps stakeholders make informed decisions. Presenting comparisons, benchmarks, or historical trends can also provide context and aid in the interpretation of results.
- Active Listening and Collaboration: Communication is a two-way process. Actively listening to stakeholders’ perspectives, understanding their needs, and engaging in collaborative discussions fosters effective communication. By incorporating feedback and addressing concerns, data scientists can build trust and ensure that their message is well-received.
- Documentation and Reporting: Clear and concise documentation of data analysis methodologies, assumptions, and results is essential for transparency and reproducibility. Writing reports, creating dashboards, and documenting code help in conveying the findings to stakeholders who may not be present during presentations.
- Ethical Considerations: In data science communication, it is important to be transparent about the data sources, any biases or limitations, and potential ethical implications. Being aware of privacy concerns and adhering to ethical guidelines builds trust with stakeholders and ensures responsible use of data.
Effective communication and storytelling in data science help bridge the gap between technical expertise and practical decision-making. By presenting insights in a compelling and understandable way, data scientists can drive understanding, engagement, and action from their stakeholders.
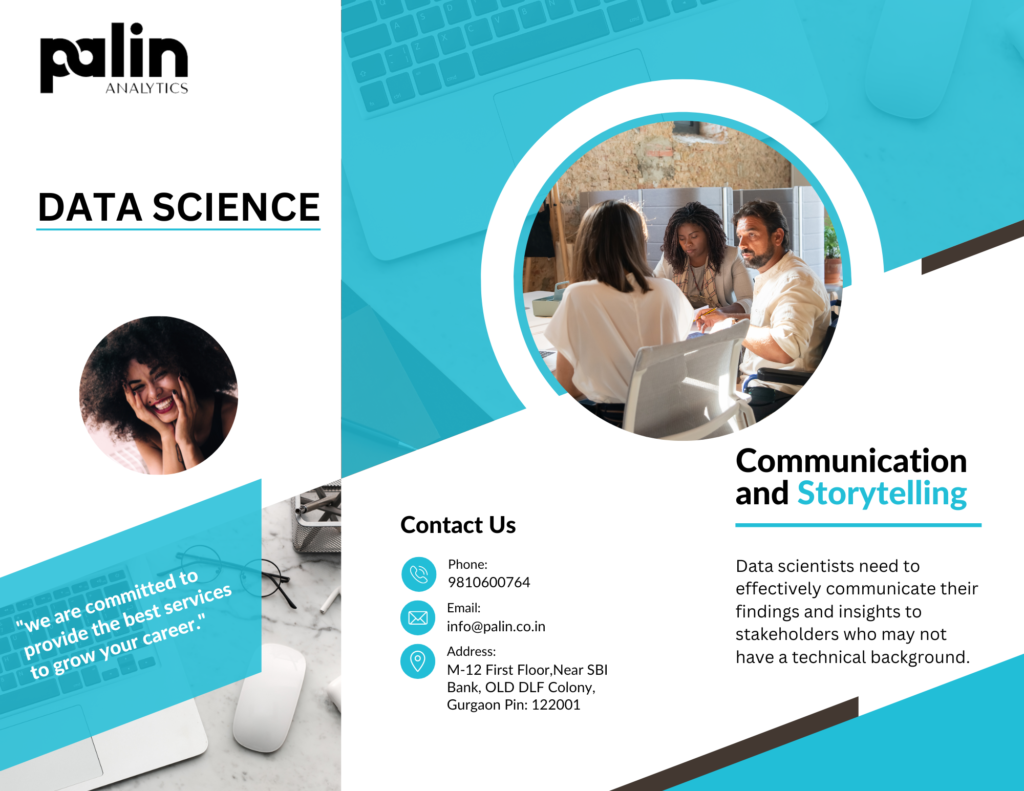