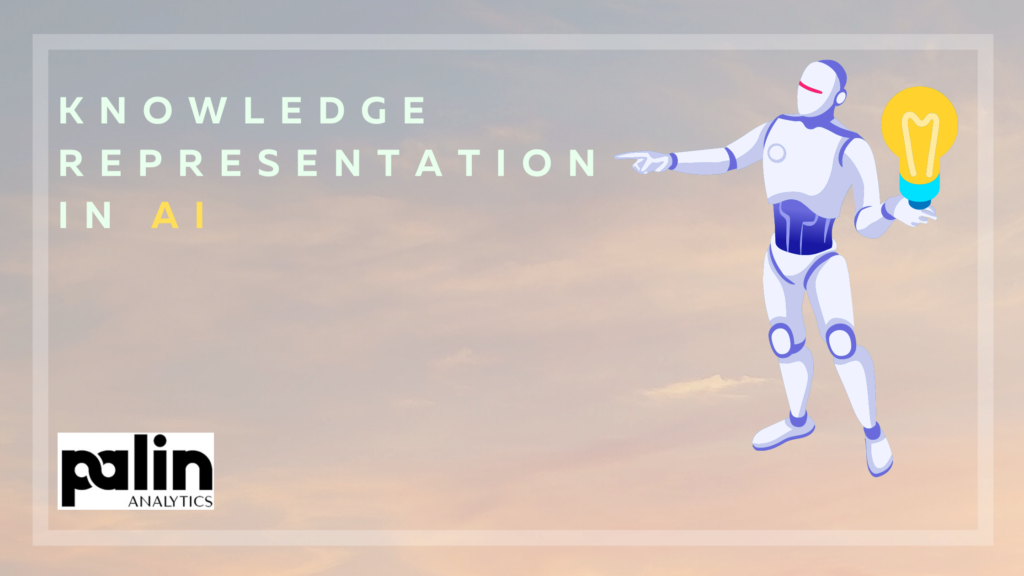
Humans excel at comprehending, thinking, and interpreting information. Humans have knowledge of things and use that knowledge to perform different acts in the real world. However, information representation and reasoning deal with how computers do all of these things. As a result, the following is a summary of knowledge representation:
- Information representation and reasoning (KR, KRR) is a branch of artificial intelligence that studies how AI agents think and how their thought influences their actions.
- It is in charge of portraying information about the real world in such a way that a machine can comprehend it and use it to solve complex real-world problems like diagnosing a medical condition or interacting with humans in natural language.
- It’s also a way of describing how artificial intelligence should reflect information. Information representation is more than just storing data in a database; it also allows an intelligent computer to benefit from its knowledge and experiences in order to act intelligently like a person.
In Artificial Intelligence, Knowledge Representation refers to the idea of identifying ways to provide machines with the knowledge that humans possess so that AI systems can improve. It is a universal truth that the more a person knows about an issue, the more likely they are to take the correct action or make the correct decision. This gives AI developers a challenge to complete in their search to make AI systems smarter: to reflect human intelligence in a way that machines can understand, and to make AI systems smarter to solve complex real-world problems. The issue is that humans process knowledge in a very complicated way.
Intuition, intentions, prejudices, beliefs, judgments, common sense, and other concepts are completely alien to machines, while some knowledge is straightforward, such as knowing some facts, general knowledge about objects, events, people, academic disciplines, and language, among other things that machines have been able to comprehend with some success. We now need to represent this information in a machine-understandable format with Knowledge Representation and Reasoning (KR, KRR) to make the AI system truly intelligent. Awareness will be defined as providing and storing environmental information, reasoning will be defined as deducing this stored information, and intelligence will be defined as making decisions and taking actions based on knowledge and reasoning.
Issues of Knowledge Representation
We discuss building a knowledge representation scheme that can represent the different types of knowledge mentioned above whenever knowledge representation in AI is discussed. This device must exhibit certain characteristics that will aid us in evaluating it. These characteristics are as follows:
- Adequacy of Representation
One of the most important properties of an information representation system is that it is adequate and capable of making an AI system understand, i.e., reflect all of the knowledge needed to deal with a specific field or domain.
- Adequacy of Inference
The knowledge representation method is adaptable enough to deal with current knowledge while making space for newly acquired information.
- Efficiency of Inference
The representation method will not be able to accommodate new information in the presence of old knowledge, but it will be able to add it quickly and seamlessly.
- Acquisition Effectiveness
The ability of the information representation system to learn new knowledge automatically will be its final property, allowing the AI to add to its current knowledge and thus become smarter and more efficient.
Knowledge representation is the secret to making future AI systems even smarter than they are now. Certain artificial intelligence knowledge representation problems must also be considered when developing a knowledge representation scheme. Significant issues include deciding which framework to use for storing information. In order to accurately reflect a subject matter, we must also consider the depth of detail needed. These are some of the variables to consider when developing such information systems.
We spoke about the different forms of information we have, how a knowledge representation system can be useful; how it can be generated and matched to it, and how knowledge can be processed. We hope for a stronger and more advanced information representation scheme in the future. One can provide knowledge to AI systems in a seamless manner; similar to how we provide knowledge to other humans, if not better.